Hedge funds worry about the legal risks of using “alternative” data
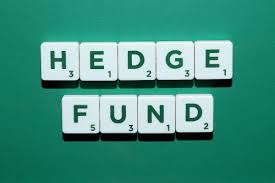
“QUANT” (quantitative) hedge funds, which craft elaborate algorithms to make trading decisions, rely on access to information. That used to mean market data, such as prices and trading volume. But some now seek an edge in novel sources. An industry has sprung up to serve them with, and help them analyse, “alternative” data, such as those gleaned from satellite images or by scraping websites. Many of these data firms have been founded by entrepreneurs, but some quant funds themselves are getting involved. Winton, a large London-based fund, is spinning off Hivemind, a data-analysis unit. A full-time management team was announced on June 18th.
For funds making macroeconomic bets by trading in, say, currencies or government bonds, real-time measures of inflation (scraped from e-commerce sites) or trade flows (from shipping data) can be better and more timely than the output of national statistics agencies. Funds trading in individual firms’ shares can infer information on sales from satellite photos of their car parks, and on footfall in shops from data bought from mobile-phone and credit-card companies, rather than having to rely on company reports or quarterly earnings statements. Many of these datasets are fine-grained. Quandl, a data provider, sells information on the number of Tesla cars sold each day, broken down by each American state.
But amid the new wave of data vendors are some that are pushing the boundaries of legality. John Funge of Winton’s San Francisco office says some are careless about privacy. Anoosh Lachin of Aspect Capital, another London quant fund, was once offered data by a former employee of the American government, who founded a firm to “predict” the statistics released by the agency he had worked for. Jonathan Streeter of Dechert, a law firm, says hedge funds are waking up to the risks of potentially suspect data. The main pitfalls are privacy laws and insider-trading rules.
The biggest risk is reputational; only egregious transgressions are likely to lead to penalties. In America, a conviction for insider trading requires not only proof that the information is material and non-public, but also proof of a “breach of duty”; that it was obtained without the owner’s consent, for example. Since many phone and credit-card companies include clauses in their contracts allowing them to sell information, that condition is rarely fulfilled. In Europe, though no breach of duty is needed to prove insider trading, the bar is higher in other ways. But privacy is a much greater concern. A new EU-wide data-protection law is backed by hefty fines.
Some funds are seeking ways to explore new datasets without breaching privacy. In a pilot project intended eventually to feed into its trading algorithms, Winton has worked with researchers at the University of California, Berkeley, to use “differential-privacy” techniques to analyse datasets that Winton was wary of looking at alone. Differential privacy works by adding noise to data, thus obscuring personal, identifiable information without destroying the dataset’s useful features. It is already used by tech firms including Google and Apple, and by America’s Census Bureau.
The nascent industry is cleaning up its act, too. Emmett Kilduff of Eagle Alpha, an alternative-data provider, points to the Investment Data Standards Organisation, a non-profit body set up earlier this year. It is unsurprising that firms such as Eagle Alpha and Quandl are moving into analysis, rather than merely providing raw data. Amid the proliferation, the need to sort useful from pointless, and legal from dubious, has never been greater.
This article appeared in the Finance and economics section of the print edition under the headline “Wary scouts”