How the Future of Artificial Intelligence Chips Will Affect Nvidia
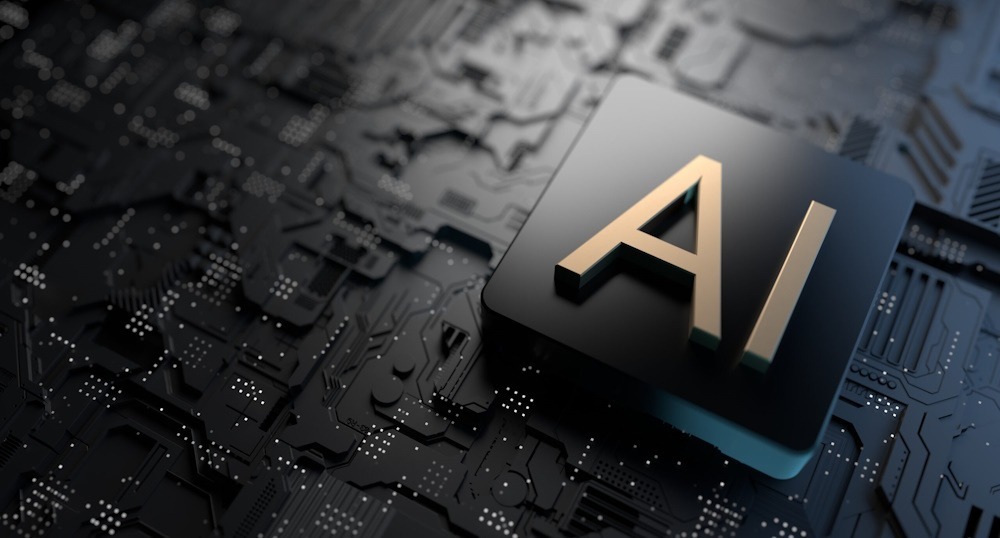
Even though Nvidia has been dominant in the AI chip market, the focus is changing to a new front that promises to be both bigger and more competitive.
Nvidia became a $2 trillion corporation by providing the hardware needed for the extremely complex task of training AI algorithms. The more chance there is to sell chips that enable those models to operate after training, producing text and images for the ever-increasing number of businesses and individuals utilizing generative AI technologies, the faster the sector will progress.
Right now, that change is helping Nvidia achieve record sales. This week, Nvidia’s chief financial officer Colette Kress stated that, out of the company’s data center business, over 40% was devoted to AI system deployment rather than training in the prior year, when revenue surpassed $47 billion. That proportion marked the beginning of a discernible change.
A few were worried that Nvidia’s dominance in the AI boom would be jeopardized by the move toward chips for installing AI systems, specifically processors that do what is known as “inference” work, but Kress’s remarks put those fears to rest.
“There is a sense that Nvidia’s share will be smaller in inferencing vs. training,” noted Ben Reitzes of Melius Research in a letter to investors. “This discovery provides more evidence that it can reap the benefits of the impending inferencing explosion.”
The increasing importance of inferencing chips has led many competitors to assume they have a leg up in the artificial intelligence market.
Intel, a maker of CPUs for data centers, anticipates rising demand for its chips as businesses strive to reduce the operational costs of artificial intelligence models. Since inferencing already makes extensive use of Intel’s specialty chips, having Nvidia’s state-of-the-art and more costly H100 AI chips isn’t absolutely necessary.
Intel CEO Pat Gelsinger stated in a December interview, “The economics of inferencing are, I’m not going to stand up $40,000 H100 environments that suck too much power and require new management and security models and new IT infrastructure.” So put it. “Assuming I can execute those models on regular [Intel] processors, it’s an easy decision.”
Bank of America analyst Vivek Arya said that the most noteworthy development to come out of Nvidia’s quarterly earnings report on Wednesday was the company’s move toward inference. The report beat Wall Street expectations, which caused Nvidia’s stock to rise 8.5% for the week and put the company’s valuation at about $2 trillion.
Following a boom in investment to train AI models, Arya predicted that inference would increase as attention turned to making money from the models. Unlike AI training, where Nvidia has a stranglehold, that might be a more competitive market.
Inference might be expanding at a quicker rate than previously thought. According to UBS analysts, 90% of chip demand is driven by training. However, by next year, that inference will only account for 20% of the market. Inference accounting for over 40% of Nvidia’s data center sales was “a bigger number than we would expect,” according to the analysts’ analysis.
It appears that Nvidia’s 80% market share in AI processors isn’t being seriously threatened just yet, according to Wednesday’s financial reports. The need for Nvidia’s chips, which are used to train AI systems, is anticipated to be high for the time being.
When developing AI systems, businesses feed massive amounts of data into their models to train them to mimic human speech in their predictions. Graphics processing units (GPUs) from Nvidia are a good fit for the massive computing power needed by the task.
Lighter lifting is inferential labor, which involves asking those models to process fresh bits of data and reply.
A number of AI-chip companies might potentially gain traction when inference becomes the main focus, joining Nvidia’s more established competitors like Intel and Advanced Micro Devices.
SambaNova’s chief executive, Rodrigo Liang, stated, “We’re seeing our inference use case exploding.” The business manufactures AI chips and software that can simultaneously perform inferencing and training. “I need to find alternate solutions because people are starting to realize that inferencing is going to be more than 80% of the cost,” he said.
Another firm that has witnessed a boost in interest recently is Groq, which was created by Jonathan Ross, a former Google AI-chip engineer. The company’s home page featured a demo that demonstrated how quickly its inference chips could generate responses from a vast language model. The company is planning to release 42,000 chips this year and 1 million next, but according to Ross, they are looking into boosting those numbers to 220,000 this year and 1.5 million next.
According to him, one reason for the change is that many of the most sophisticated AI systems are being fine-tuned to provide better results without undergoing retraining, which means that more computational resources are being directed towards inference. Additionally, he claimed that Groq’s specialized chips outperformed Nvidia’s and other chip firms’ products in terms of runtime efficiency and cost.
“The use of inference is cost-dependent,” he stated. “A lot of the models that were trained at Google ended up not getting deployed because they were too expensive to put into production, even though they were all perfectly good.”
Recognizing the impending change and the advantages of being able to execute inference more cheaply, big IT companies like Meta, Microsoft, Alphabet’s Google, and Amazon.com have been attempting to build inference processors in-house.
For instance, inference accounts for forty percent of the computational expenses associated with Amazon’s Alexa smart assistant, according to Swami Sivasubramanian, a VP of data and machine learning at the cloud-computing arm of the corporation, who stated last year. Inference chips have been available to Amazon since 2018.
Nvidia is aiming to maintain its position as the shift toward inference continues. A new chip continued its years-long reign as industry leader with top results in a critical AI inference benchmark last year.
In a blog post dated December, Nvidia retaliated against AMD for allegedly releasing superior artificial intelligence (AI) chips in comparison to Nvidia’s inference capabilities. According to Nvidia, if AMD used optimized software to make its performance claims, their chips would be twice as fast.
Lucy Harlow
Lucy Harlow is a senior Correspondent who has been reporting about Equities, Commodities, Currencies, Bonds etc across the globe for last 10 years. She reports from New York and tracks daily movement of various indices across the Globe